Written By Rich Jiang On 9th November 2022
Face Recognition (FR) is a biometric technology that performs identity recognition based on the information of human face features. A series of related technologies that use a camera or a camera to capture an image or video stream containing a human face, and automatically detect and track the face in the image, and then perform face recognition on the detected face, are often also called portrait recognition and facial recognition.
The research of face recognition system began in 1960s, and was improved after 1980s with the development of computer technology and optical imaging technology, while it really entered the primary application stage in late 1990s; with the progress of artificial intelligence technology mainly deep learning in recent years, face recognition technology has been developed rapidly. "Face recognition system" integrates artificial intelligence, machine recognition, machine learning, model theory, expert system, video image processing and other professional technology, is a comprehensive and strong system engineering technology. At present, our all-in-one self kiosks has been very mature use of face recognition technology.
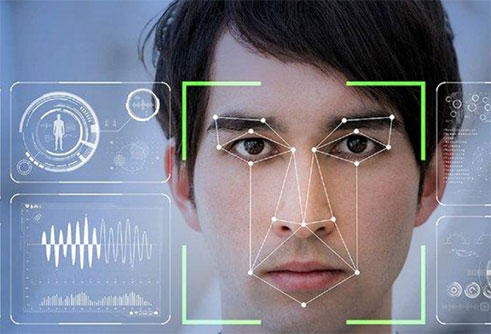
Face Recognition Process
Face recognition system usually includes several processes: face image acquisition and detection, key point extraction, face regularization (image processing), face feature extraction and face recognition comparison.
Face image acquisition. Different face images can be captured through the camera lens, such as static images, dynamic images, different positions, different expressions and other aspects can be well captured. When the user is within the capture range of the capture device, the capture device will automatically search and capture the user's face image.
Face detection. Face detection is mainly used in practice for pre-processing of face recognition, i.e., to accurately calibrate the position and size of a face in an image.
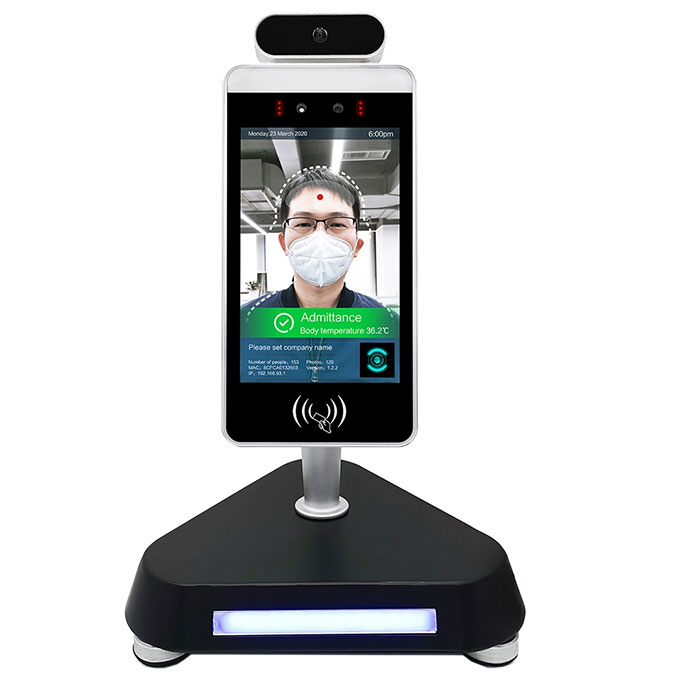
Key point extraction (Feature Extraction). The features that can be used in face recognition systems are usually classified as visual features, pixel statistical features, face image transformation coefficient features, face image algebraic features, etc. Face feature extraction is done for certain features of the face. Face feature extraction, also known as face characterization, is the process of feature modeling of a human face. The methods of face feature extraction are summarized into two main categories: one is knowledge-based representation methods; the other is representation methods based on algebraic features or statistical learning.
Face regularization (Preprocessing). Image preprocessing for faces is the process of processing images and ultimately serving feature extraction based on face detection results. The original image acquired by the system is often not directly usable due to various conditions and random interference, and it must be subjected to image preprocessing such as grayscale correction and noise filtering in the early stage of image processing. For face images, the pre-processing process mainly includes light compensation, grayscale transformation, histogram equalization, normalization, geometric correction, filtering and sharpening of face images.
Face recognition comparison (matching and recognition). The extracted feature data of the face image is searched and matched with the feature template stored in the database by setting a threshold value, and when the similarity exceeds this threshold value, the result obtained by matching is output. Face recognition is to compare the features of the face to be recognized with the obtained face feature template, and to judge the identity information of the face according to the degree of similarity. It can be divided into 1:1, 1:N, and attribute recognition. Where 1:1 is to compare the eigenvalue vectors corresponding to 2 faces, 1:N is to compare the eigenvalue vector of 1 face photo with the eigenvalue vector corresponding to another N faces, and output the faces with high similarity or top X similarity ranking.
Face Feature Analysis Algorithm
It combines computer image processing technology and biostatistical principles in one, using computer image processing technology to extract human image features from the video, and using biostatistical principles to analyze and establish a mathematical model, i.e. face feature template. The completed face feature template is used to analyze the features with the human face of the subject, and a similarity value is given according to the result of the analysis. This value is used to determine whether the face is the same person. At present, we have face recognition technology applied to advertising machines to serve customers more efficiently, such as: Face recognition attendance kiosk, face recognition payment kiosk machine, face recognition temperature measurement kiosk, etc.
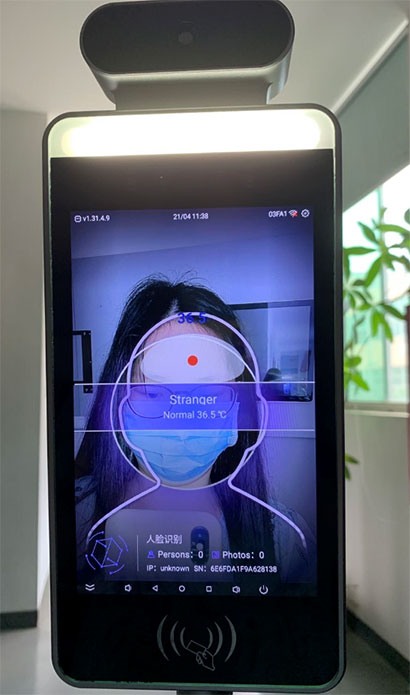
3D Face Recognition Technology
3D face recognition technology is an important development discovery of face recognition. At present, the scope of most face recognition applications is limited to 2D images. The human face is essentially a three-dimensional model, and 2D face recognition is susceptible to factors such as posture, lighting, and expression because 2D images themselves have a defect in that they cannot represent depth information well. If deep learning is to understand face recognition from the perspective of human cognition, then 3D technology is to reflect face recognition from a reality model.
The current algorithm research on 3D face recognition direction is not as rich and deep as 2D face recognition technology, and many factors limit the development of this technology. First, 3D face recognition often requires specific acquisition equipment, such as 3D cameras or binocular cameras. Currently such acquisition devices are still relatively expensive and are mainly used for specific scenarios. Second, the 3D modeling process requires a large amount of computation and has high hardware requirements, which also limits the current application. Third, the 3D face recognition database is relatively sparse, and researchers lack training samples and test samples to carry out more in-depth theoretical research. It is believed that with the future development of chip technology and sensors, when the computational power no longer receives constraints and the cost of 3D acquisition equipment drops significantly, 3D face recognition will make an important breakthrough.
Rich Jiang
Rich Jiang is the Marketing Director at CY Digital Signage. And he has over 15 years experiences in digital signage displays.